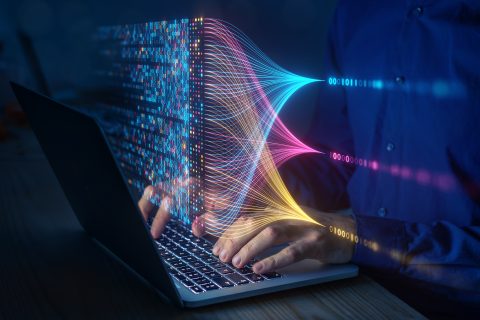
Building better AI models for the railways starts with a solid data foundation
The railway industry is rapidly digitalising and adopting new technologies such as machine learning and artificial intelligence (AI). These new technologies allow for increased overall efficiency in the sector, which is instrumental in reaching sustainability goals in Europe and mitigating the existing strain on the labour force. Dr Igor Dakic, Senior Consultant in Strategic Asset Management at the supplier of engineering, design, and advisory services AFRY, discusses the challenges companies wishing to utilise their data to train AI models for instance, are facing.
Want to read more?
You have read all of your free premium articles for this month. Please become a subscriber to keep reading.
Subscribe now!
Take advantage of our exclusive offer to get full access to all premium content.